According to International Data Corporation, spending on artificial intelligence (AI) and machine learning is estimated to grow from $12 billion in 2017 to $57.6 billion by 2021.
It’s the future, and people who make that happen are data scientists. Some workers such as Xavier Conort saw it coming early on and had jumped into action to get a spot in the thriving industry.
The French national spent the first 10 years of his career as an actuary assessing the pricing and risks of insurance policies though data.
However, Xavier left his job at the age of 40 in 2011 after taking up an interest in machine learning. Seeing the potential of how technology could help his line of work and beyond, he embarked on a quest to master it, while offering consultancy services on the side.
Today, the Singapore Permanent Resident is the chief data scientist at DataRobot, whose automated machine learning platform has penetrated seven cities worldwide, including Singapore.
Decoding Machine Learning
Machine learning is a field of computer science developing AI that self-learns and improves through data and algorithms.
It sounds complicated, and that is why data scientists out there have built platforms like DataRobot for companies that do not have the expertise to use.
Simply put, the application breaks down large volumes of complex data into something that can be recognised, analysed and automated. The end user will receive reports with predictions or trends that are useful to the business.
Xavier explained: “Typically, the statistician will remove insignificant variables from the data before analysis, but it is impossible to do so manually when you have hundreds of thousands of variables to check. That is where the regularisation mechanism in the machine comes in to help and enable you to work with more data. Sometimes when people talk about big data, it is referring to the large quantity of information that a machine is able to digest.”
Getting There
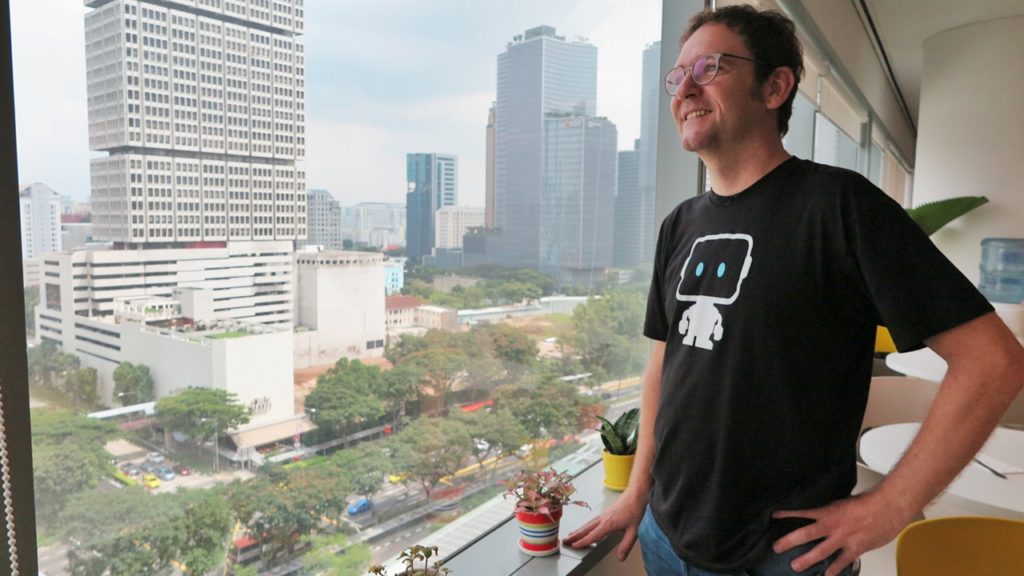
To pick up the necessary skills back then, Xavier often ploughed through open-source resources, as well as published works and online courses by professors in data science.
Here are some of his recommendations:
What he found the most useful was Kaggle, an international live competition platform where he could put what he learned into practice. He won about a third of the 30 competitions he participated.
“Data scientists love to solve problems, but nobody talks about data science projects because companies are very secretive. So, Kaggle is very successful in creating an online community where data scientists can share, challenge and improve ideas,” shared 45-year-old Xavier.
Makings of a Data Scientist
Driven by the industry demand for machine learning, data scientists are highly sought after.
Started in 2013, DataRobot currently has built a 400-strong team globally, of which 150 are data scientists and engineers. The company currently has 25 employees based in Singapore and plans to double that by 2019.
The use of machine learning is wide and varied, such as:
- Banking: Predict loan defaults or fraud
- Retail: Improve supply chain
- Human Resource: Suggest interventions to lower staff attrition rate
- Insurance: Shorten time taken for repetitive tasks involved in price analysis and medical claims processing
- Sales & marketing: Identify ways to up-sell and cross-sell
- Healthcare: Forecast occupancy of hospital beds
- Sports: Help coaches track performance of individual players
A report by Gartner estimates such analytics by artificial intelligence will generate some $1.3 trillion in business value this year.
Debunking that technical knowledge is all it takes on the job, Xavier referred to data science as a form of art that requires plenty of passion and soft skills. These include:
- Understanding of coding and its best practices
- Creativity to mine new types of data
- Common sense to ask the right questions
- Know how to convert business problems into data problems